Expert presents novel approach at prestigious computational research conference
June’s NAACL conference will see machine learning specialists from technology company Iprova present a paper introducing a new and effective method for the unsupervised training of machine learning algorithms to infer sentence embeddings. The NAACL (North American Chapter of the Association for Computational Linguistics) Human Language Technologies (HLT) conference will take place at the Hyatt Regency New Orleans hotel, Louisiana, from June 1-6 2018.
The research paper, entitled “Unsupervised Learning of Sentence Embeddings using Compositional n-Gram Features”, will be presented by Matteo Pagliardini. Pagliardini is a senior machine learning engineer at Iprova and one of the three scientists that authored the research paper and developed the new model for unsupervised training, Sent2Vec. The other authors are Prakhar Gupta and Professor Martin Jaggi of EPFL (École polytechnique fédérale de Lausanne).
Sent2Vec forms part of Iprova’s pioneering technology that provides a data-driven approach for the creation of commercially relevant inventions. Hundreds of patents have been filed based on Iprova’s inventions by some of the world’s most respected technology companies.
While there have been several successes in deep learning in recent years, the paper notes that these have almost exclusively relied on supervised training. Pagliardini cites a specific research paper by Mikolov et al (2013) as being particularly worthy of note for the success of semantic word embeddings — representations of words with similar meanings — trained unsupervised. The new paper presents a way of finding similar success for longer sequences of text rather than individual words.
“There are very useful semantic representations available for words but producing and learning semantic embeddings for longer text has always proven difficult”, explained Pagliardini. “It was especially challenging to see whether such general-purpose representations could be obtained using unsupervised learning.”
“By taking inspiration from the existing C-BOW model of the Word2Vec algorithm, we were able to develop a computationally efficient method to train sentence embeddings. Our evaluations found that our method achieves a better performance on average than most other models, with a particular proficiency in evaluating sentence similarity. At NAACL HLT, we will explore our research further and explain where future work may take our Sent2Vec model.”
The paper was accepted for the NAACL HLT conference after an extensive review process from leading figures in the computational research community. The Sent2Vec model outlined in the paper is open source and available for use.
Those unable to attend NAACL HLT 2018, or interested in finding out how to use the technology can visit the company’s website and view the research paper.
More from our feed
Iprova featured in IEEE Spectrum magazine
Read more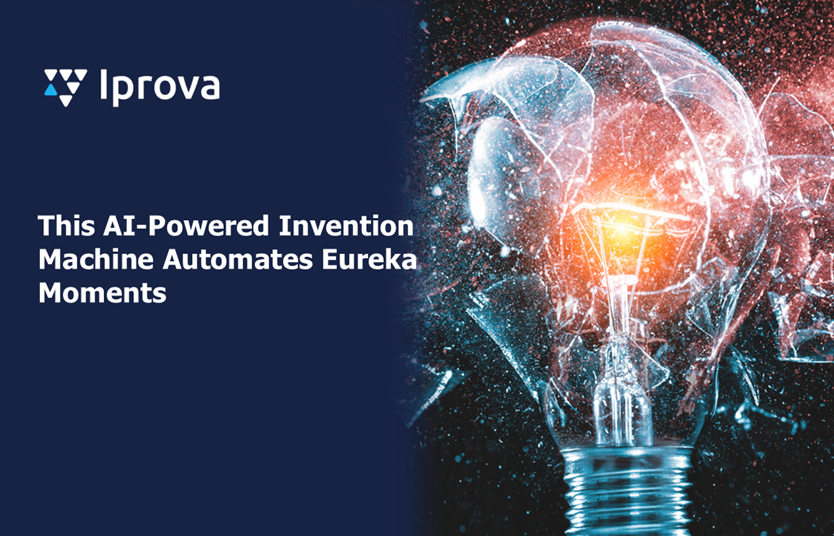
AI invention tool sees patent surge
Read more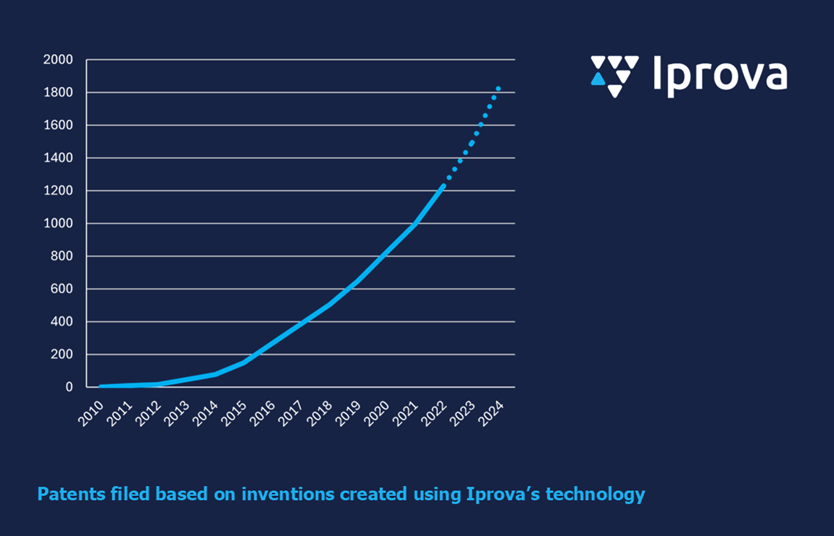
Iprova enhances ground-breaking invention platform with powerful discovery and analysis tool
Read more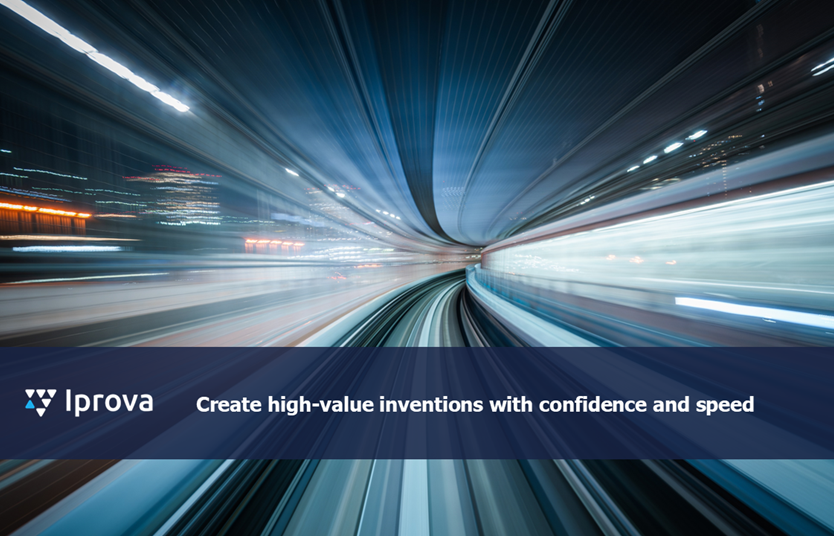